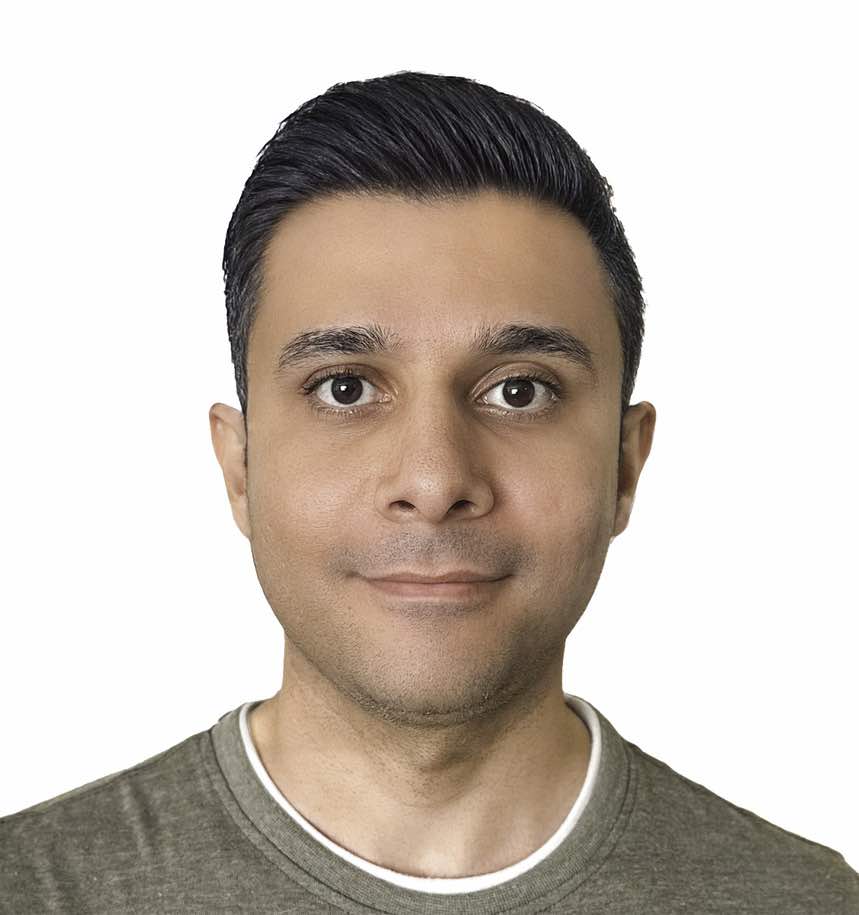
My passion lies at the overlap of Human-Computer Interaction (HCI) and Artificial Intelligence (AI) and the challenge of integrating the two fields. Currently, I'm pursuing my Ph.D. at the University of Pittsburgh's School of Computing and Information, under the guidance of Dr. Peter Brusilovsky, head of the PAWS Lab.
My research focuses on building and evaluating search and recommender systems that are user-centric and intelligent. I design solutions that integrate AI algorithms with user-friendly interfaces, effectively bridging the gap between high-level AI technologies and their practical applications. This approach underlines my commitment to making advanced technologies accessible and beneficial for everyone in their everyday contexts.
Having been fortunate to receive support from esteemed institutions such as National Institutes of Health (NIH), National Science Foundation (NSF), National Library of Medicine (NLM), and Amazon Research Awards (ARA) among others, my work is a testament to the potential of combining UX principles with the robustness of AI. These projects range from design and implementation of multiple online systems for healthcare (addressing IBD, ovarian cancer, and aphasia) and education (involving student advisement, e-readers, and the exploration of academic and medical documents), as well as more theoretical research on probabilistic models and simulation-based evaluation of interactive recommender systems.
The fusion of AI and HCI offers a promising horizon, and I am enthusiastic about the advancements we can achieve in this domain. With every research project and publication, I am committed to pushing this frontier, ensuring technology is more aligned with its users.
Read more
Education
-
► Ph.D. in Information Science
2019 - NowUniversity of Pittsburgh, Pittsburgh, USA
Thesis: Human-AI Collaboration in Search and Recommendation
-
► M.Sc. in Computer Science and Engineering
2014 - 2017Polytechnic University of Milan, Milan, Italy
Thesis: Event-Based User Profiling in Social Media Using Data Mining Approachesn
Experience
► Applied Scientist Intern, AWS AI Labs
Activities: Developing an end-to-end LLM-Based framework for generating personalized ,robust and factually correct explanation for recommendations by utilizing fine-tuning, prompt engineering and chain-of-thought techniques.
► Graduate Research Assistant
Activities: Conducting theoretical and experimental research on Interactive recommender systems. Implementing several online search and recommender systems for experiments and public use. Publishing and presenting in top-tier conferences. directing graduate and undergraduate research assistants.
► Research Fellow
Activities: Conducting research on data-driven fashion and travel. Analyzing huge amounts of social media data to find patterns related to various fashion and tourism related events. Collaborating with designers in the school of design to build customized fashion entity recognition models.
► Senior Software Developer
Activities: Managing a small software development team focusing on building web apps for small business financing. Communicating with other teams to find optimal solutions for similar problems. Meeting directly with customers to understand their requirements.
► Software Developer
Activities: Building custom web solutions for organizations and businesses as a full-stack developer. Communicating with customers and other stakeholders. Leading the UX team for the last 6 months.
► Software Developer
Activities: Working as a java developer building computer software for a variety of customers.

Publications
Title | Project/Research | Year |
---|---|---|
![]() Balduini, M., Brambilla, M., Della Valle, E., Marazzi, C., Arabghalizi, T., Rahdari, B., & Vescovi, M. Big Data Research |
User Modeling in Smart City | 2019 |
![]() Rahdari, B., Brusilovsky, P., & Babichenko, D. Hypertext '20 |
Grapevine | 2020 |
![]() Rahdari, B., Brusilovsky, P., Babichenko, D., Littleton, B., Patel, R., Fawcett, J., Blum, Z. ASSIS&T '20 |
Grapevine | 2020 |
![]() Rahdari, B., Brusilovsky, P., Thaker, K., & Barria-Pineda, J. ECTEL '20 |
Reading Mirror Integeration | 2020 |
![]() Rahdari, B., Brusilovsky, P., Thaker, K., & Barria-Pineda, J. iTextbooks '20 |
Reading Mirror Integeration | 2020 |
![]() Rahdari, B., Brusilovsky, P. IUI '20 |
Paper Tuner | 2019 |
![]() Rahdari, B., Arabghalizi A., & Brambilla M. Springer |
Social Media Analysis | 2017 |
![]() Arabghalizi, T., Rahdari, B., & Brambilla, M. WEB-KD |
Social Media Analysis | 2017 |
![]() Rahdari, B., & Brusilovsky, P. KDD '19 Workshop |
Grapevine | 2019 |
![]() ![]() Rahdari, B., Brusilovsky, P., & Javadian Sabet, A. RecSys '21 |
Grapevine | 2021 |
![]() Tsai, C. H., Brusilovsky, P., & Rahdari, B. IUI '19 Workshop |
Paper Tuner | 2019 |
![]() Rahdari, B., Brusilovsky, P., Thaker, K., & Chau, H. K. epiDAMIK '20 |
Covex | 2020 |
![]() Rahdari, B., & Arabghalizi, T. Master Thesis |
Soacial Media Analysis | 2016 |
![]() Rahdari, B., Kveton, B., & Brusilovsky, P. Hypertext '22 |
Interactive RecSys | 2022 |
![]() Rahdari, B., & Brusilovsky, P. IUI '21 Workshop |
Paper Explorer | 2021 |
![]() ![]() Rahdari, B., Tsai, C. H., & Brusilovsky, P. FLAIRS '19 |
Paper Tuner | 2019 |
![]() Rahdari, B., Brusilovsky, P.. He, D., Thaker, K., Luo, Z., Lee, Y. RecSys '22 |
HELPeR | 2022 |
![]() Rahdari, B., Brusilovsky, P., & Kveton, B. FLAIRS '22 |
Interactive RecSys | 2022 |
![]() Rahdari, B., Brusilovsky, P., & Sabet, A. J. RecSys '21 Workshop |
Grapevine | 2021 |
![]() Dmitriy Babichenko, Behnam Rahdari, Ben Stein, Suraj Subramanian, Claudia Ramos Rivers, Gong Tangand David Binion BIOSTEC 2022 |
IBD DSS Tool | 2022 |
![]() Behnam Rahdari, Hao Ding, Ziwei Fan, Yifei Ma, Zhuotong Chen, Anoop Deoras and Branislav Kveton. WSDM 2024 |
Explainable RecSys | 2024 |
![]() Khushboo Thaker, Behnam Rahdari, Peter Brusilovsky, Chi Ching Vivian Hui, Zhimeng Luo, Youjia Wang Daqing He, Heidi Donovan, Young Ji Lee AMIA 2023 |
HELPeR | 2023 |
![]() Behnam Rahdari, Peter Brusilovsky and Branislav Kveton. TORS 2024 |
Interactive RecSys | 2024 |
Research
► Investigating and Evaluating Exploratory Recommender Systems
Funding Agency:Amazon Research Awards (ARA) - Partial
Remote /Pittsburgh, PA
► Integrating complementary learning principles in aphasia rehabilitation via adaptive modeling
Funding Agency:National Institutes of Health (NIH)
Pittsburgh, PA
► Health E-Librarian with Personalized Recommendations (HELPeR)
Funding Agency:National Library of Medicine.
Pittsburgh, PA
► Utilizing Clinical Metadata to Predict High-Cost Complications and Treatment Response in IBD: Development of Clinical Decision Support Tools
Funding Agency:United States Department of Defense.
Pittsburgh, PA
► It’s Who You Know”: Feasibility of a Hybrid Recommender System to Connect Students With Informal Social Networks of Pitt Researchers
Funding Agency:University of Pittsburgh - Office of the Provost.
Pittsburgh, PA
Projects
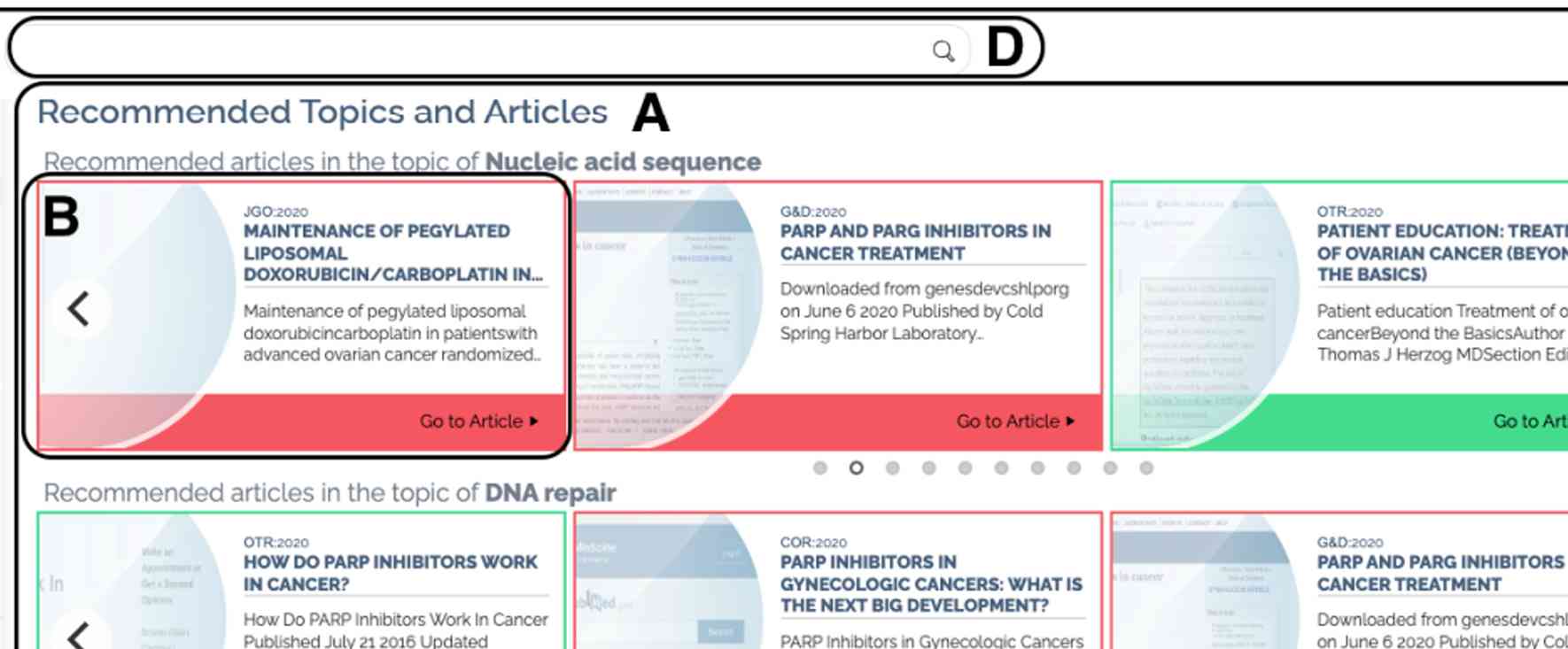
► HELPeR System
HELPeR is a personalized online resource recommendation system for ovarian cancer patients and caregivers. It adapts to users' health literacy levels and specific needs to provide relevant and accessible information.
More Details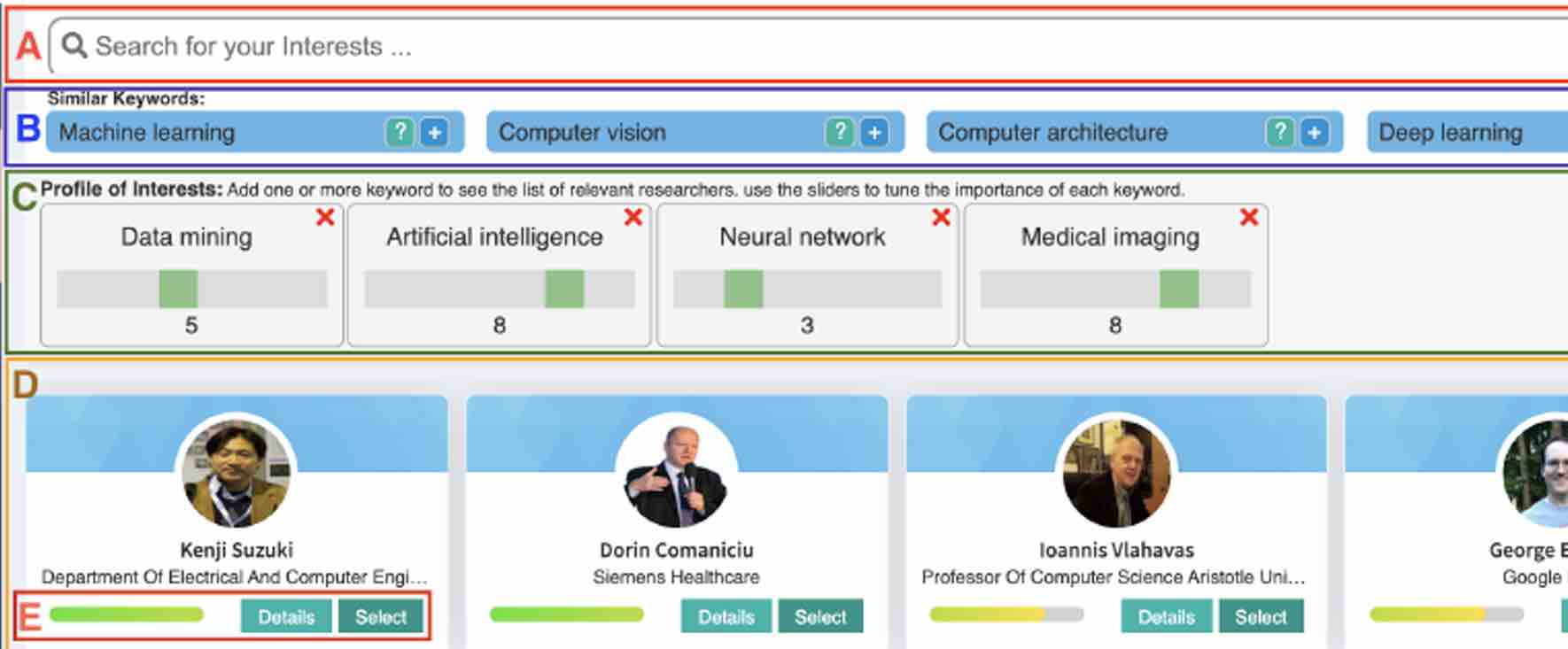
► Grapevine System
Grapevine is an exploratory search system that helps undergraduate students find research advisors by adapting to their interests and knowledge level. It uses a user-friendly interface and knowledge graph to connect students with compatible advisors.
More Details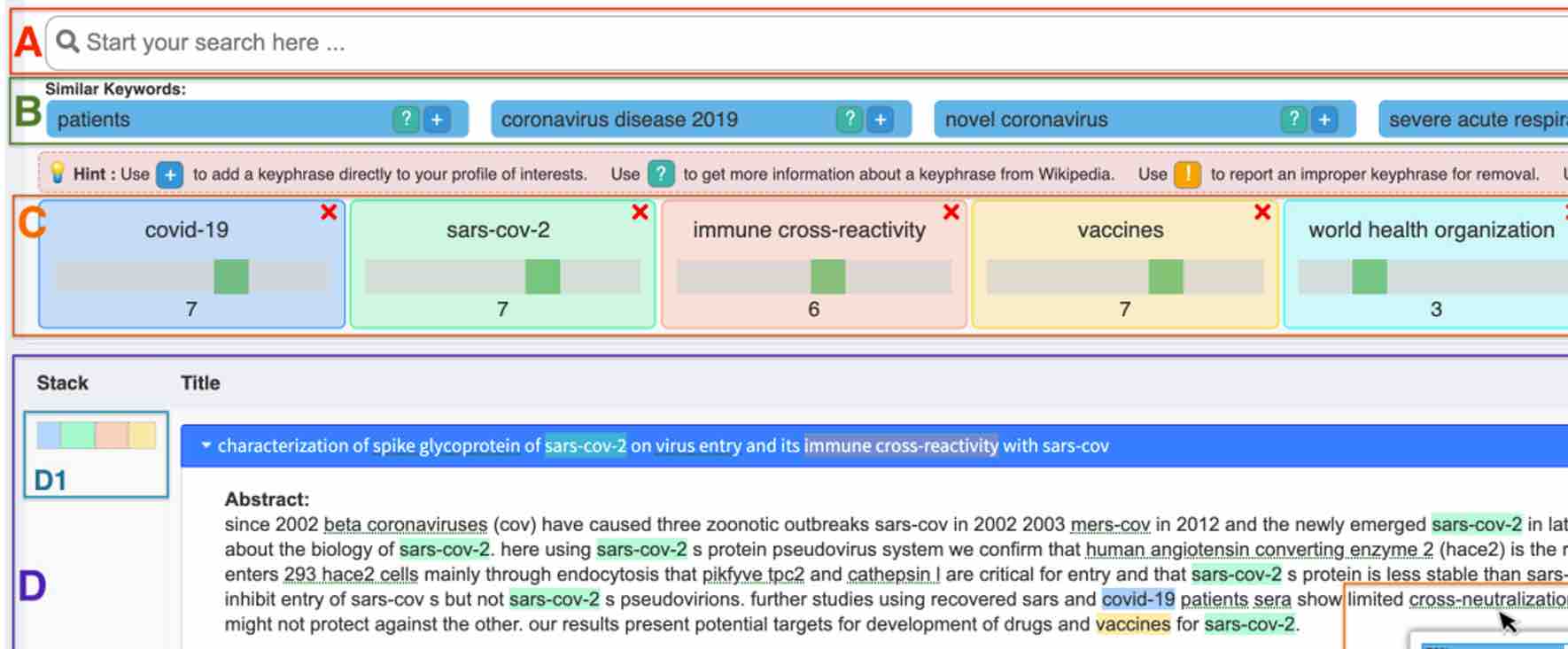
► Covex System
CovEx is an exploratory search system for COVID-19 scientific literature, offering personalized and dynamic recommendations using concept extraction knowledge graphs and user preferences.
More Details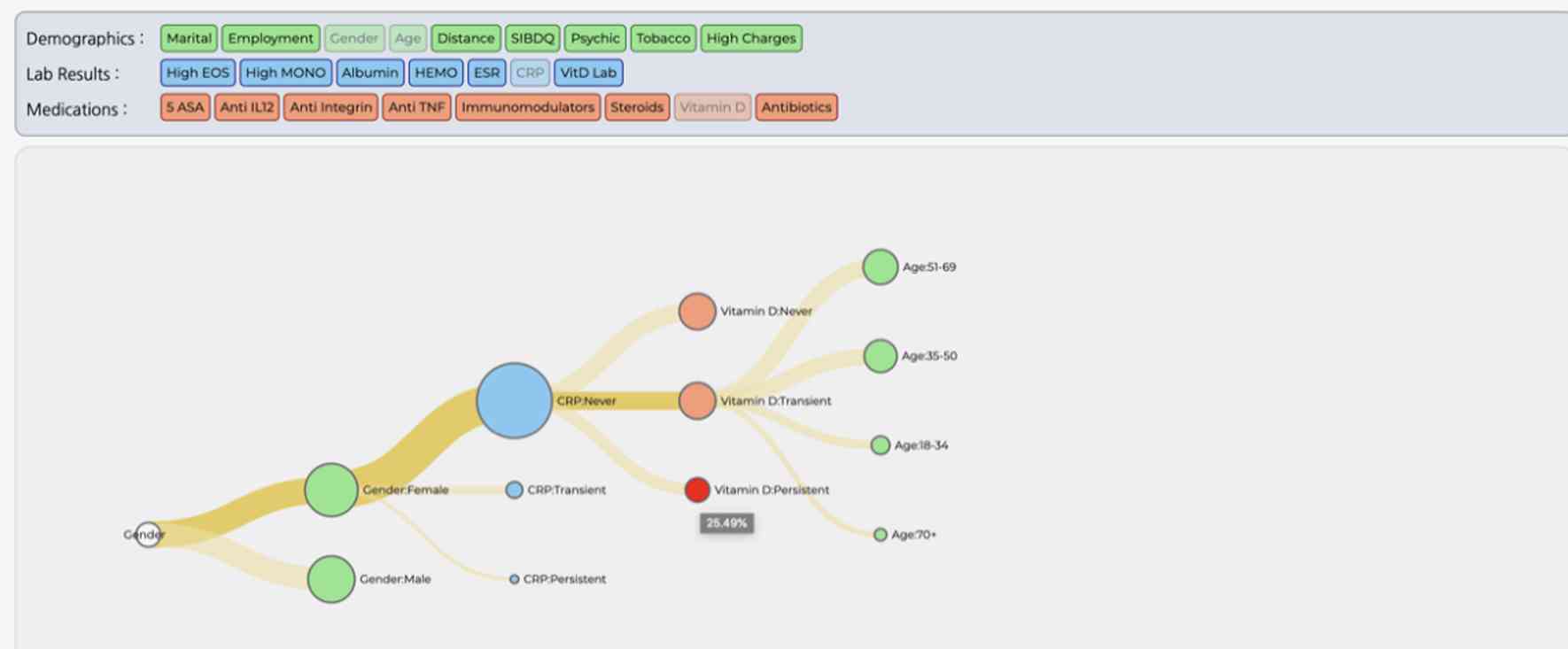
► IBD CDSS Tool
The IBD project involves developing an interactive browser dashboard, offering users a comprehensive and customizable view of information, tailored to their specific browsing needs and preferences.
More Details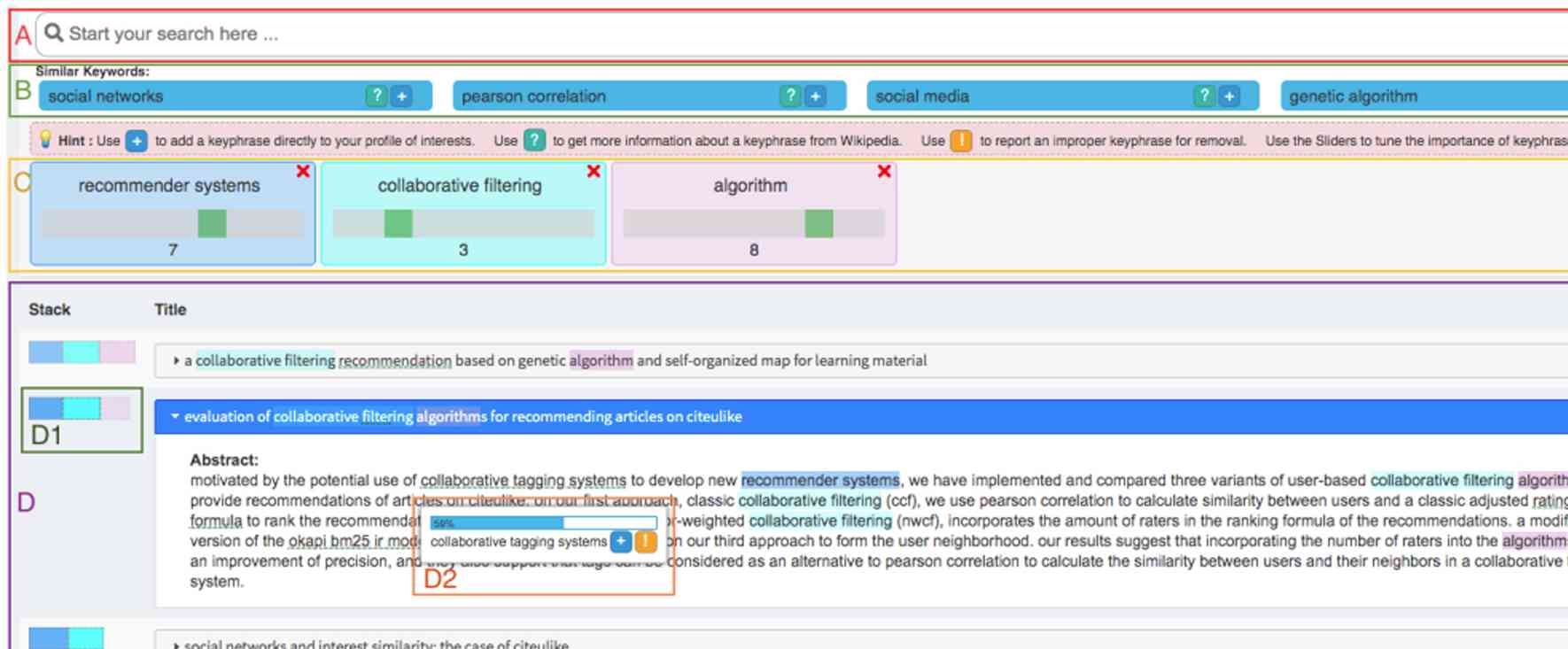
► Paper Explorer System
PaperExplorer is a system designed to enhance exploratory search in academic conference proceedings, using concept extraction knowledge graphs and user-controlled recommendation to personalize the discovery process.
More Details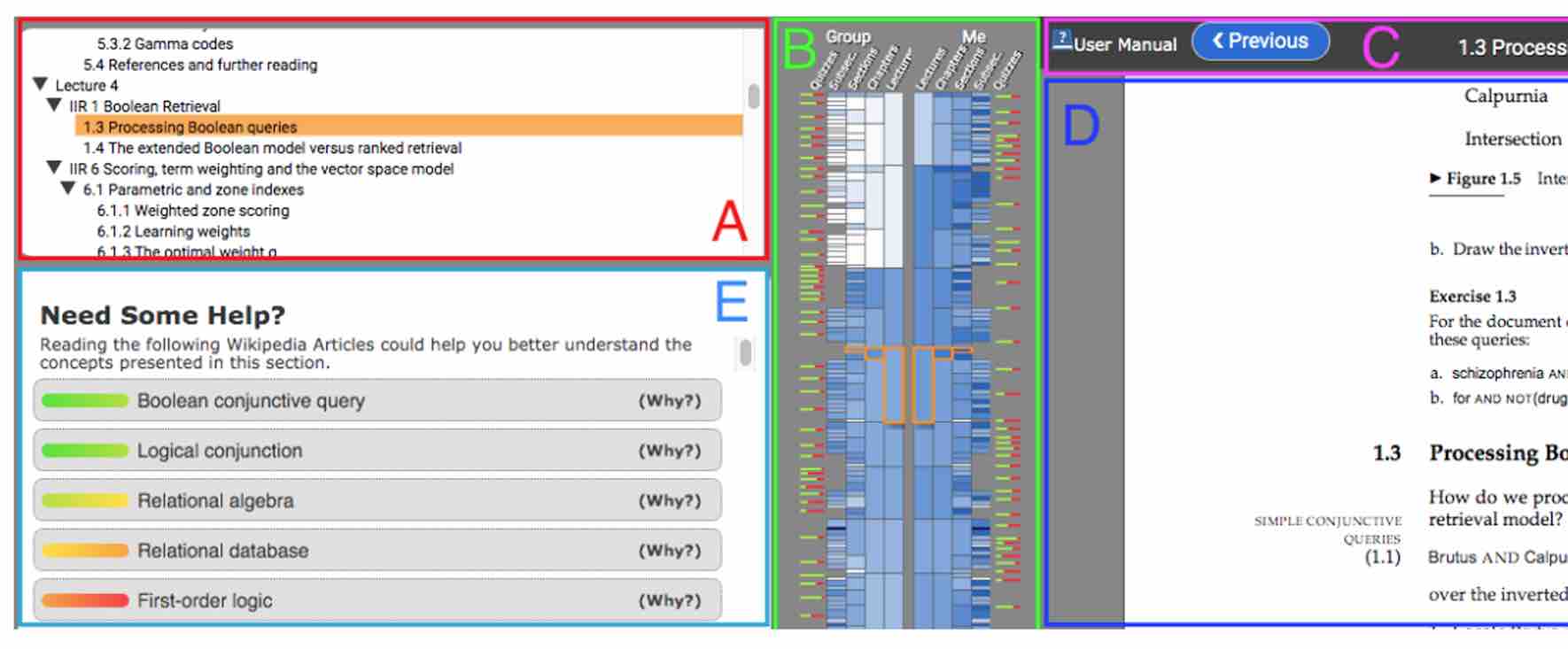
► Reading Mirror Integeration
The Reading Mirror Integration project focuses on enhancing electronic textbooks with a knowledge graph-based system, providing students with contextually relevant Wikipedia article recommendations to supplement their learning.
More Details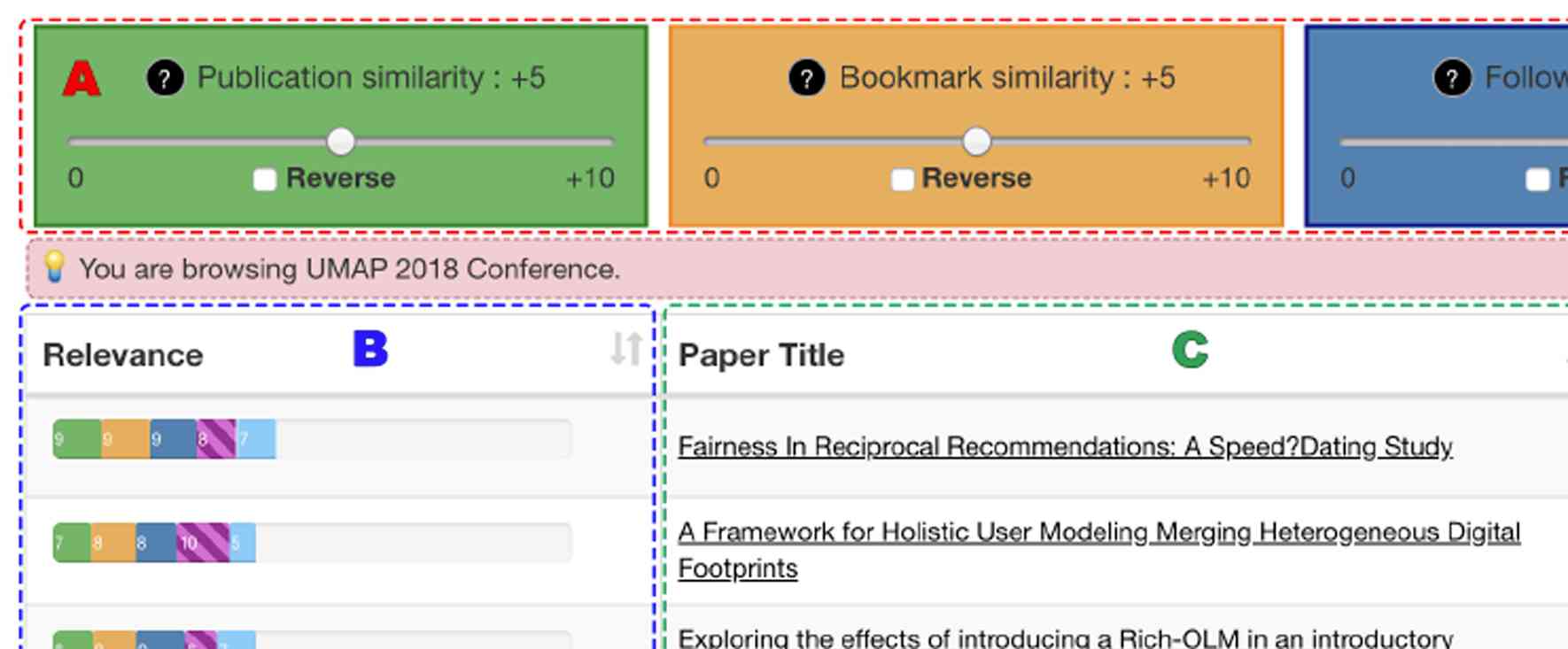
► Paper Tuner System
Paper Tuner is a user-controlled hybrid recommendation system for academic papers, allowing users to tailor search results to their preferences in conference environments.
More Details► HELPeR System
Funding Agency:National Library of Medicine
Remote / Pittsburgh, PA
Ovarian cancer, a complex and challenging disease, requires patients and caregivers to carefully review vast array of medical information to make informed health decisions. To assist in this endeavor, the project 'HELPeR: Health e-Librarian with Personalized Recommender' has been developed. This innovative system aims to empower ovarian cancer patients and caregivers by providing personalized, accessible, and up-to-date information. Recognizing the diverse health literacy levels and specific informational needs of users, HELPeR is designed to streamline the process of finding reliable, relevant, and recent resources. This system could benefit patients in a landscape where patients and caregivers often face overwhelming amounts of data, varying in quality and relevance.
At the heart of HELPeR's utility is its user-friendly interface, which allows for intuitive navigation and interaction with a vast array of resources. As depicted in Figure 1, the interface features a straightforward layout with a search bar, filtering options, and a carousel display for search results. This design is deliberately chosen to ensure ease of use and familiarity for users of all backgrounds. The system's unique feature is its ability to adapt to the user's health literacy level, highlighted by color-coded document difficulty indicators and the option to select the desired level of complexity. This personalization ensures that users are not overwhelmed by overly technical information and can access resources that match their comprehension and needs.
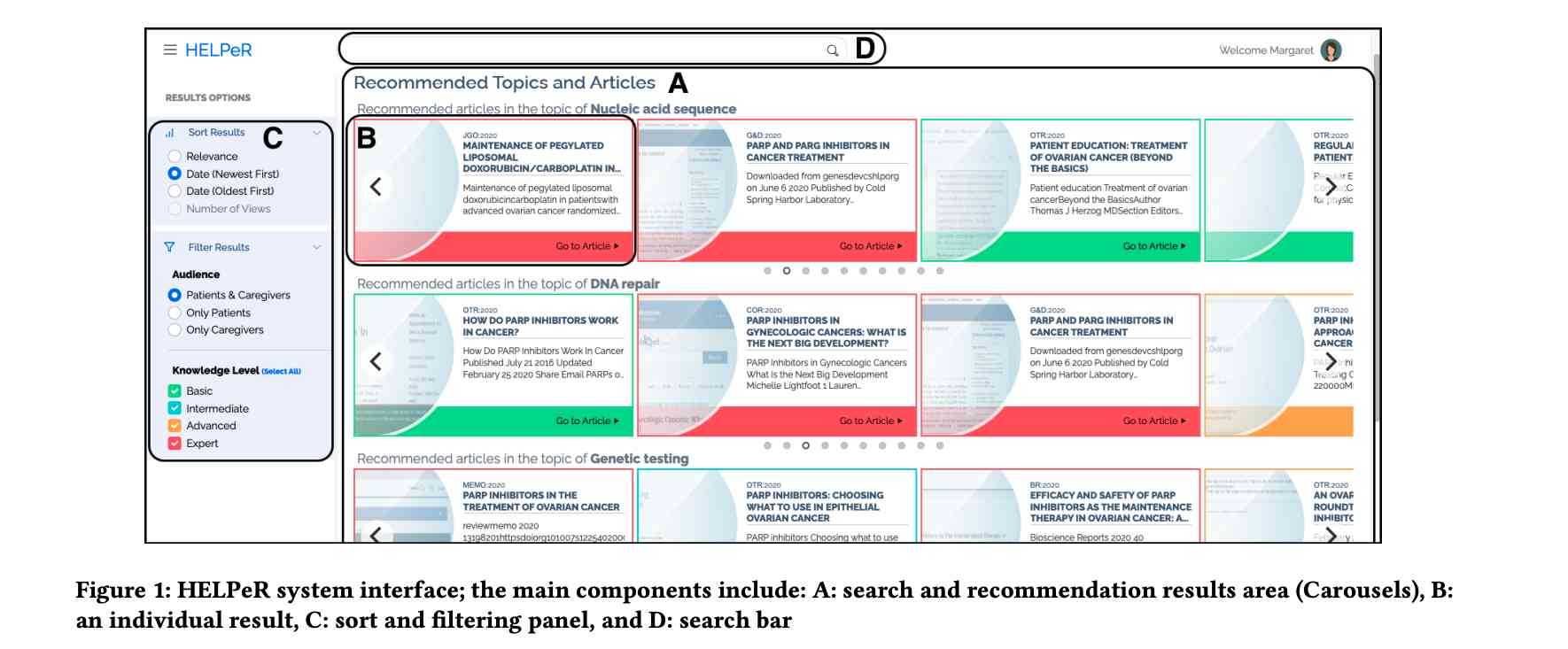
Underlying HELPeR’s user-centric interface is a sophisticated architecture, encapsulated in Figure 2, which is composed of three primary pipelines: document collection, user profiling, and presentation. The document collection pipeline is dedicated to curating current and credible information, while the user profiling pipeline leverages data from health providers and patient activities to create detailed user profiles. These profiles then inform the presentation pipeline, ensuring that the system delivers the most relevant and personalized content. Built on a native graph database, HELPeR offers quick and responsive interactions, making it an efficient tool for users seeking specific information. This system not only bridges the information gap for ovarian cancer patients and caregivers but also sets a precedent in health informatics by providing a tailored, interactive, and adaptive platform for medical information dissemination.
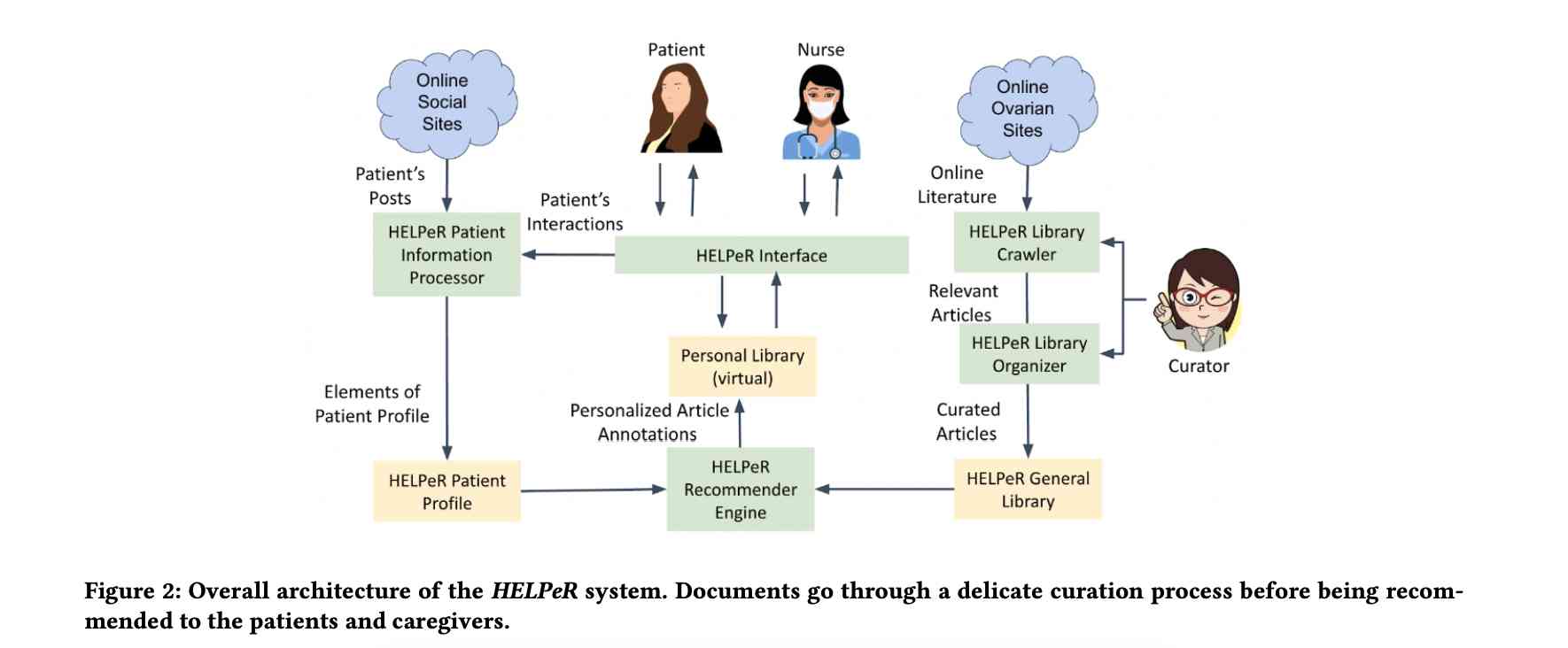
► Grapevine System
Funding Agency:University of Pittsburgh - Office of the Provost
Pittsburgh, PA
Finding a suitable research advisor is a significant challenge for undergraduate students, especially for those who are new to the intricacies of academic research. Grapevine addresses this challenge by offering a tailored system that simplifies the process of matching students with research advisors. It's particularly beneficial for first-generation college students who may not have prior exposure to navigating academic environments. Grapevine's aim is to facilitate these students' entry into the research domain, providing a supportive tool to identify advisors whose interests align with their own.
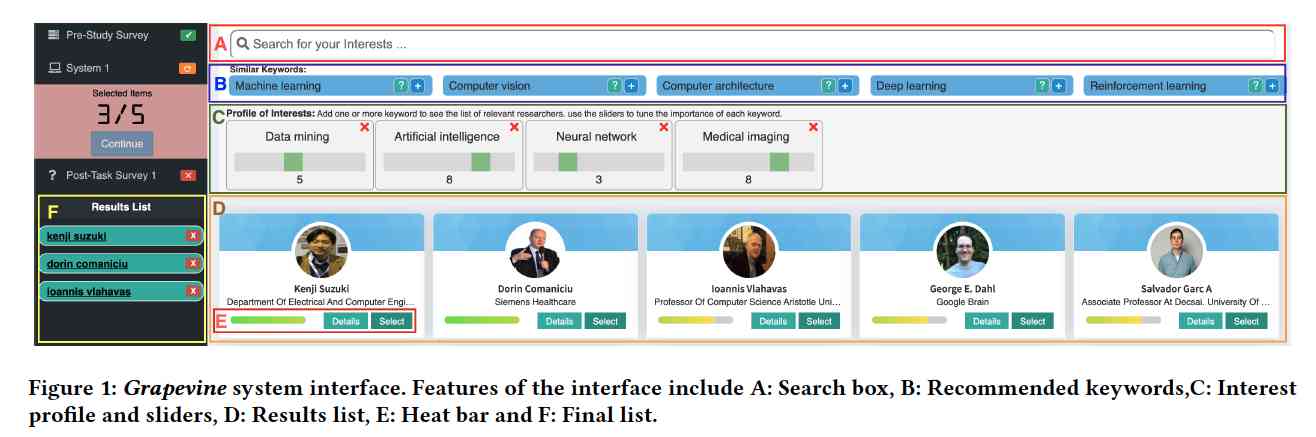
The interface of Grapevine, illustrated in Figure 1, is user-centric and intuitive. It features a search box, a list of suggested keywords, sliders for adjusting research interests, and a display of potential advisors. This design enables students to actively engage in refining their research preferences, helping them to clarify and articulate their interests more effectively. Detailed advisor profiles, as seen in Figure 2, offer in-depth information about each advisor’s research areas, assisting students in making informed decisions. The system's underlying knowledge graph, shown in Figure 3, links advisors, research topics, and keywords to generate personalized recommendations, adapting to each student's evolving research interests.
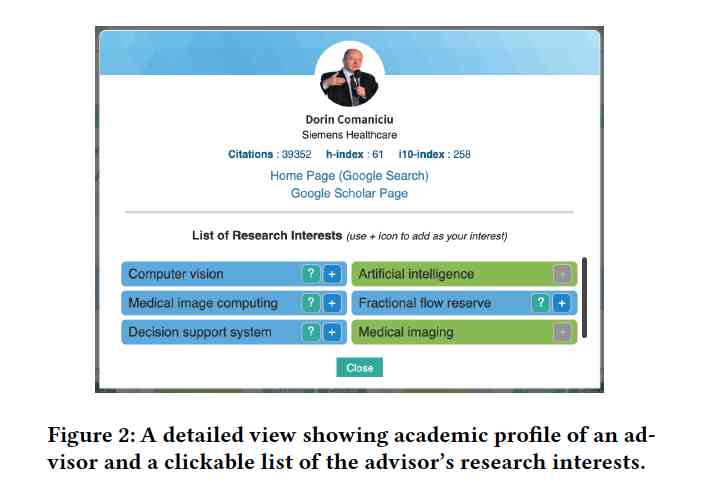
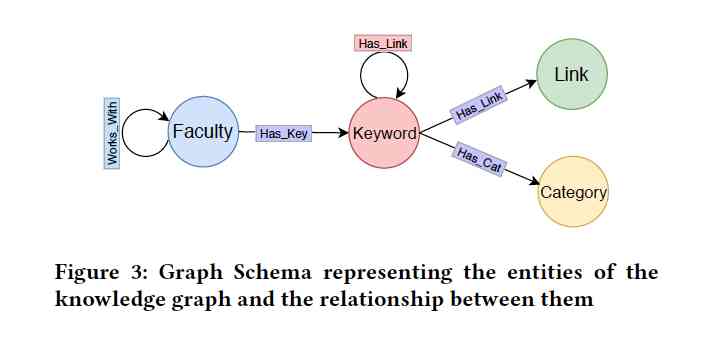
In essence, Grapevine serves as a practical and accessible platform for undergraduate students embarking on their research journeys. It's designed to demystify the process of finding a research advisor, making it more approachable and aligned with individual student needs. By leveraging technology to connect students with compatible advisors, Grapevine hopes to contribute to creating a supportive environment for the next generation of researchers.
► Covex System
Funding Agency:
This project was undertaken as a volunteer initiative without external funding
Pittsburgh, PA
Navigating the overwhelming volume of COVID-19 related scientific literature is a daunting task for researchers, medical professionals. The CovEx system has been developed to streamline this process, offering a targeted exploratory search tool for efficiently locating relevant publications. Utilizing a combination of user-controlled recommendation algorithms and a concept extraction knowledge graph, CovEx aims to simplify the task of sifting through the extensive COVID-19 research data.
The user interface of CovEx, shown in Figure 1, is crafted to be intuitive and user-friendly. It features an instant search box for starting queries, a section for exploring similar keywords, adjustable sliders for keyphrases to refine search results, and a section for displaying search outcomes. This setup is designed to allow users to continuously modify their search parameters, making the process more interactive and responsive to their specific needs. The system also offers a quick view of publication abstracts, helping users evaluate the relevance of their findings.
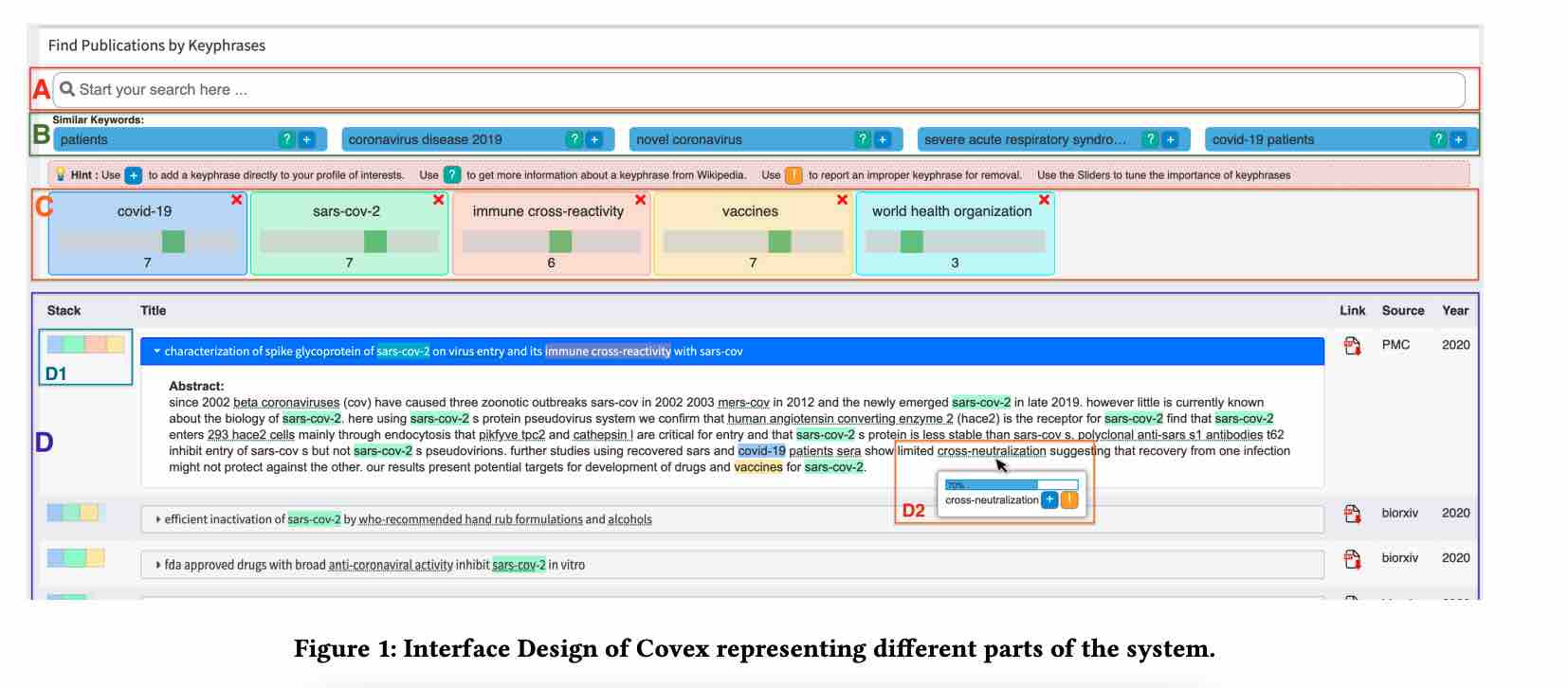
Central to CovEx's functionality is its knowledge graph, which links publications, authors, and keyphrases from the COVID-19 Open Research Dataset Challenge (CORD-19). This graph supports the discovery of relevant literature by leveraging the relationships between these various elements. CovEx's search process involves initially selecting candidate publications and then reordering them based on their relevance to the user’s profile. This approach focuses on delivering personalized and pertinent search results.
In essence, CovEx serves as a tool to assist in managing the influx of information related to COVID-19 research. It offers a platform that prioritizes user preferences and needs in the search process, aiming to make the exploration of scientific literature more manageable and focused.
► Paper Explorer System
Funding Agency:
University of Pittsburgh
Pittsburgh, PA
In the realm of academic research, one of the key challenges is efficiently navigating through the dense and expansive world of conference proceedings to find relevant literature. PaperExplorer is a system developed to tackle this challenge, aiming to assist users in the exploratory search of academic papers. Its primary objective is to provide a more intuitive and tailored search experience for a diverse range of users, from seasoned researchers to individuals who are newer to the field.
PaperExplorer's approach to enhancing the search experience revolves around the creation of a user interest profile. This profile is built using keyphrases that users select to reflect their research interests. Unlike traditional search engines that rely on full keyphrase queries, PaperExplorer supports the incremental discovery and refinement of interests, allowing users to steer their search process. The interface, as depicted in Figure 1, includes a straightforward search box, an area for recommended keyphrases, and a results section, all designed to facilitate an interactive and dynamic exploration of academic content.
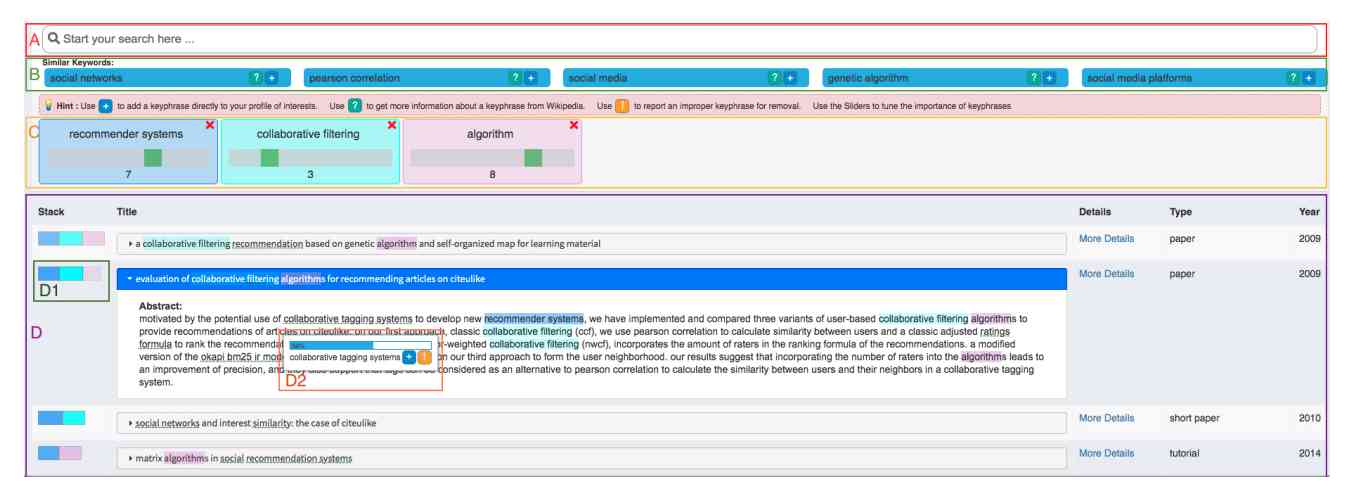
At the core of PaperExplorer's functionality is a knowledge graph, which links publications, authors, and keyphrases, derived from a dataset of conference proceedings. This graph is essential for the system's recommendation process, enabling it to provide suggestions that are closely aligned with the user's defined interests. The search process in PaperExplorer involves first selecting candidate publications based on the knowledge graph and then reordering these publications in accordance with the user’s interest profile. This methodology is aimed at delivering search results that are not only relevant but also personalized to each user's specific research needs.
PaperExplorer is currently in its developmental phase, being refined and tested for its capability to improve the exploratory search process in academic settings. The project represents an effort to provide a solution to the complexities of searching through academic conference literature, striving to make this process more user-centric and efficient.
► Paper Tuner System
Funding Agency:
University of Pittsburgh
Pittsburgh, PA
Finding relevant academic papers in conference proceedings can be challenging, particularly when navigating through a vast array of topics and research areas. To address this, we developed Paper Tuner, a system designed to enhance the process of discovering academic literature. Paper Tuner is a hybrid recommendation system that offers a more personalized approach to academic searches, enabling users to tailor their exploration according to their individual research interests and preferences.
The main feature of Paper Tuner is its user-controlled interface, which allows for the adjustment of various recommendation sources. This interface, as seen in Figure 1, includes sliders for each source, enabling users to modify the weight of these sources in their search results. Additionally, the interface displays stacked relevance bars next to each result, providing a visual representation of how each source contributes to the overall recommendation. This level of user control is a key aspect of Paper Tuner, allowing for a more dynamic and user-specific search experience compared to traditional static recommendation systems.

In Paper Tuner, we incorporated several recommendation sources, such as publication similarity, bookmark similarity, followee similarity, and author popularity, to create a comprehensive recommendation system. One innovative feature is the reversible relevance mechanism, which lets users reverse the impact of certain sources, offering even more customization in the search results. This system was put to the test at the EC-TEL 2018 conference, where it was used by authors and attendees. The feedback from this real-world application was encouraging, with users actively engaging with the customization features, demonstrating the practical utility of Paper Tuner.
In developing Paper Tuner, our aim was to provide a solution that makes the search for academic papers more focused and user-centric. By allowing users to control their search process, we hope to enhance the efficiency and effectiveness of academic literature discovery, especially in the context of conference proceedings.
► Reading Mirror Integeration
Funding Agency:
University of Pittsburgh
Pittsburgh, PA
The rapid expansion of digital learning resources presents a unique challenge for students using electronic textbooks: how to effectively navigate and utilize these resources to supplement their textbook content. To address this, we developed a recommendation system that integrates a knowledge graph to suggest relevant Wikipedia articles to students. This system is designed to enhance the learning experience by providing students with external content that complements their textbook studies and aligns with their current knowledge level and interests.
The interface of our recommendation system, depicted in Figure 1, integrates seamlessly with electronic textbooks (see section E). It automatically suggests relevant Wikipedia articles, offering students alternative or foundational resources that enhance their understanding of textbook content. These recommendations are tailored to each student’s learning stage and are triggered based on their interactions within the textbook, such as starting new units or responding to embedded questions.
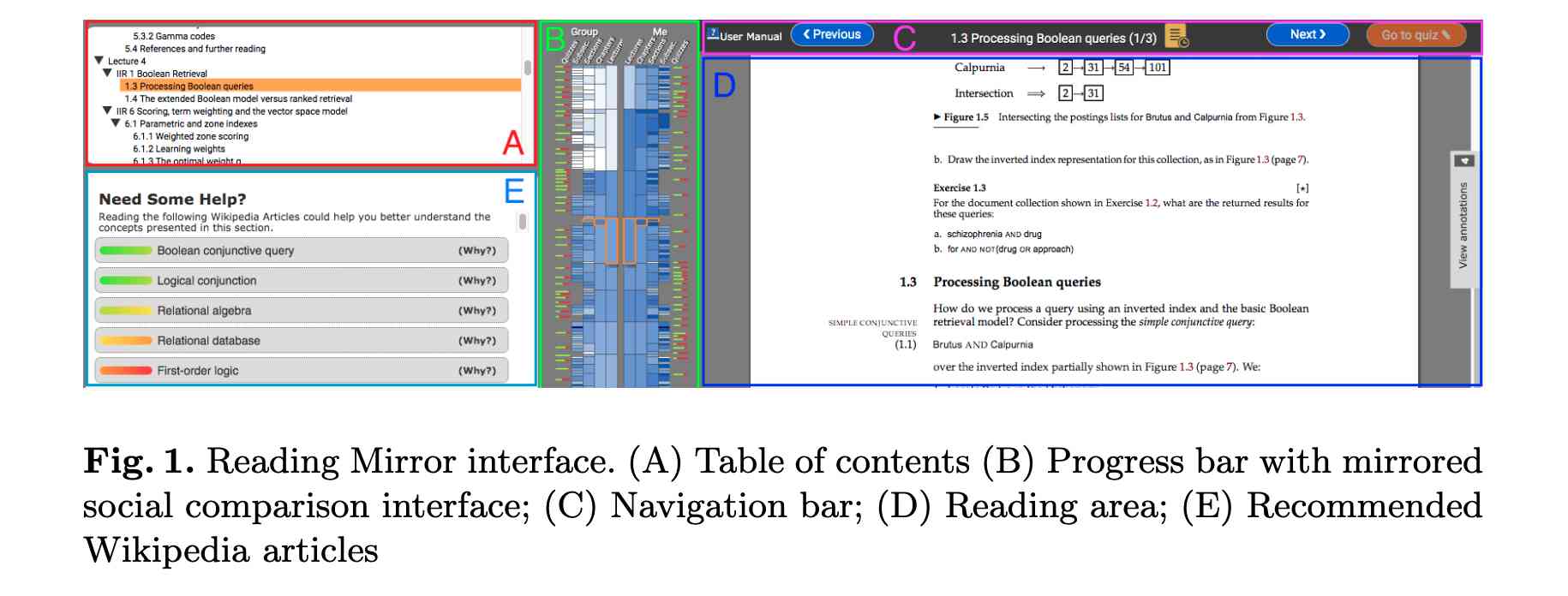
A unique feature of our system, illustrated in Figure 2, is its capability to provide explanations for each recommendation. This functionality helps students understand the relevance of the suggested articles to their current textbook content. The underlying technology of this recommendation system is a knowledge graph, shown in Figure 3. This graph connects textbook content, Wikipedia articles, and the student's learning profile, forming the basis for our personalized recommendation approach.
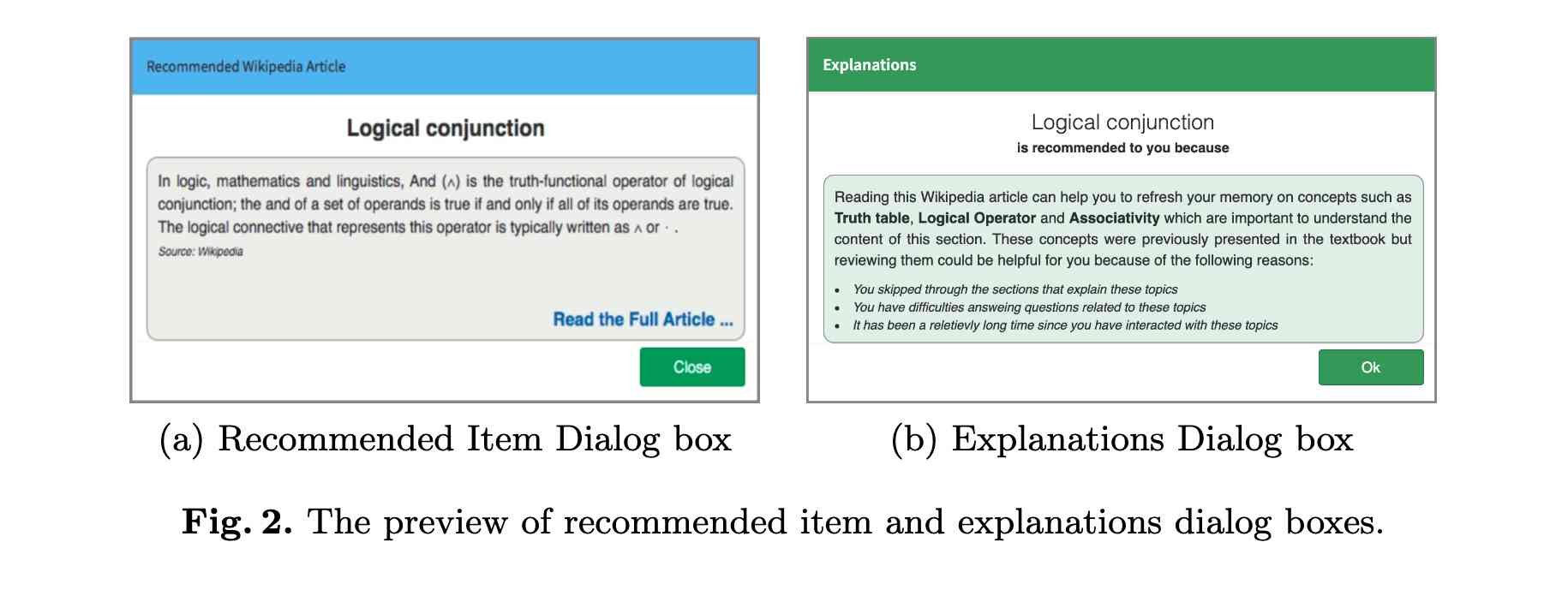
By integrating this knowledge graph-based system into electronic textbooks, our goal is to streamline students' access to supplementary resources. This system not only directs students to relevant external content but also provides context for why these resources are beneficial to their learning journey. Through this project, we aim to enhance the digital learning experience, making it more intuitive, informative, and tailored to individual student needs.
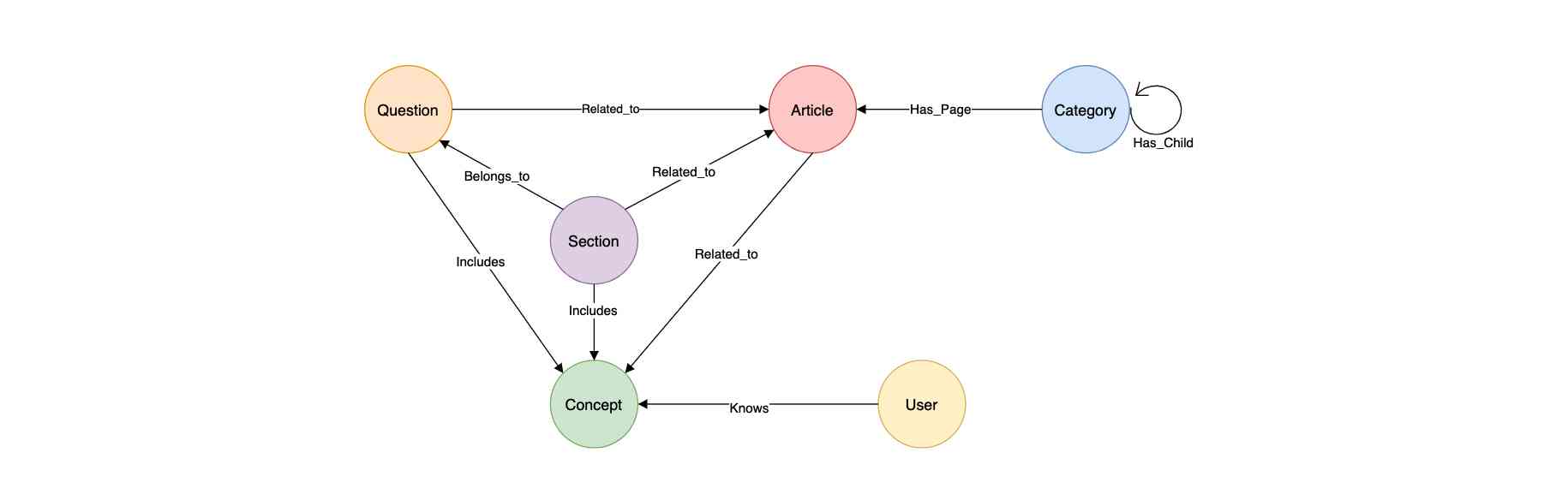
► IBD Clinical Decision Support Tools
Funding Agency:United States Department of Defense
Pittsburgh, PA
Since their inception in the early 1980s, Clinical Decision Support Systems (CDSS) have significantly evolved, transitioning from basic rule-based systems to sophisticated, machine learning-driven platforms for diagnostic assistance. This evolution over three decades has closely linked the trust clinicians place in CDSS with their understanding of the system's design and the inner workings of its algorithms.Clinicians' trust in Clinical Decision Support Systems (CDSS) is significantly influenced not just by their confidence in using these systems but more crucially by the depth and clarity of the explanations provided about the diagnostic processes.
On the other hand, there's a concern of 'automation bias,' where clinicians might trust the system too much, leading to the acceptance of incorrect suggestions from the CDSS.
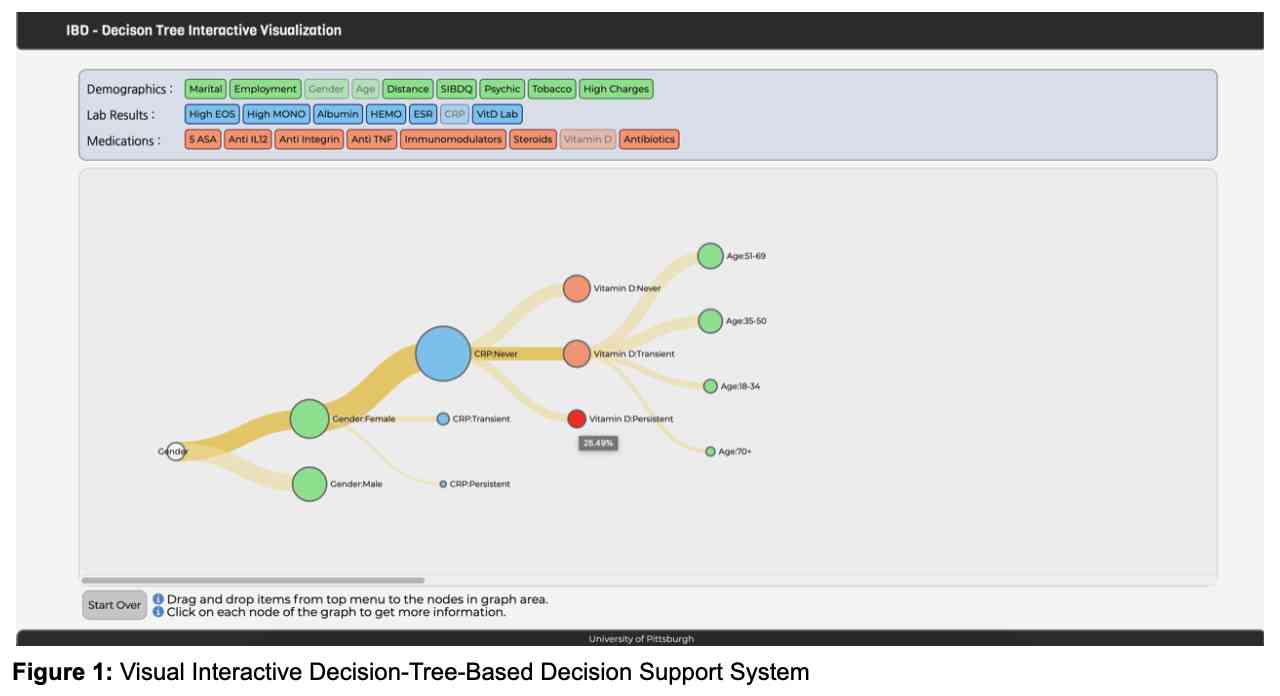
In this project. we desgined and developed a visual decision support system (DSS) (Figures 1 and 2) that allows IBD clinicians to compare an individual patient’s disease progression and responses to treatments with those of a larger patient cohort. Such comparison allows clinicians to identify the most likely disease progression and response to treatments for a given patient.
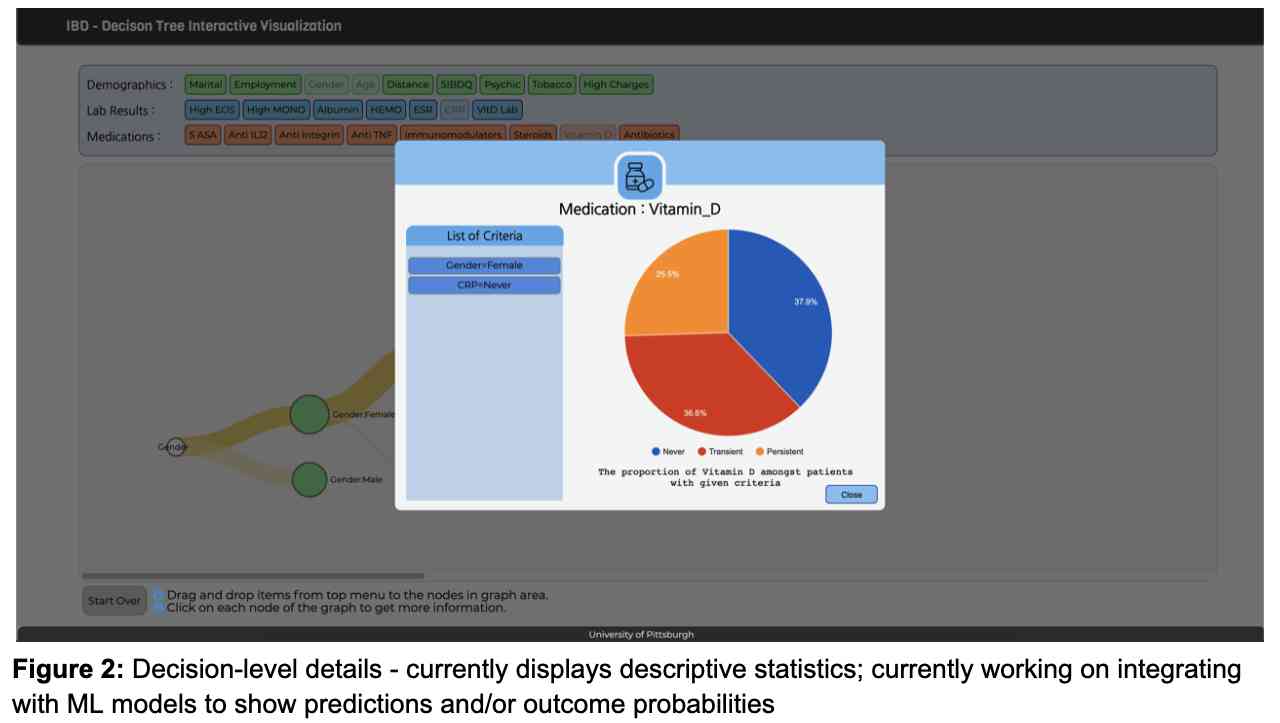
We hypothesize that this interactive visual CDSS will reduce the cognitive load and time-to-treatment-plan compared to traditional patient chart reviews in the Epic Electronic Medical Records (EMR) system. Our interactive CDSS provides visualizations of treatment pathways for different cohorts and makes it easy for clinicians to understand how and why the CDSS made its suggestions, which we believe will foster a high level of trust in this decision support mode. Figure 3 showcases the new tree-based visual interface with uniformly sized nodes and links.
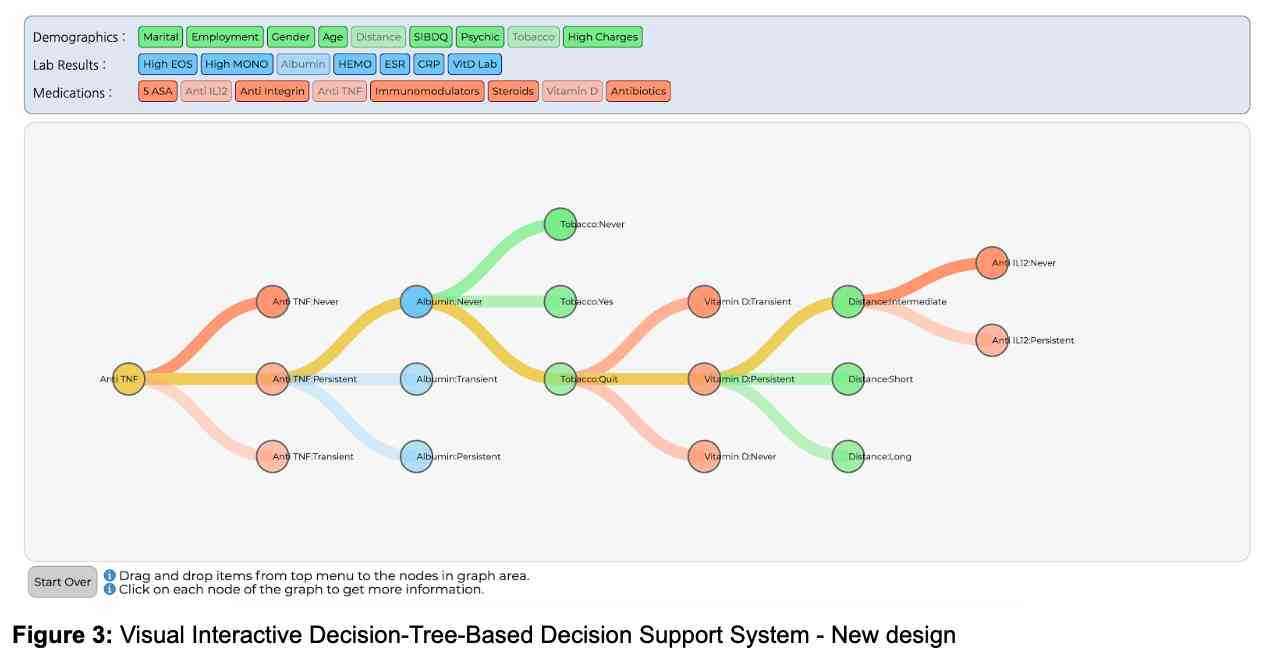